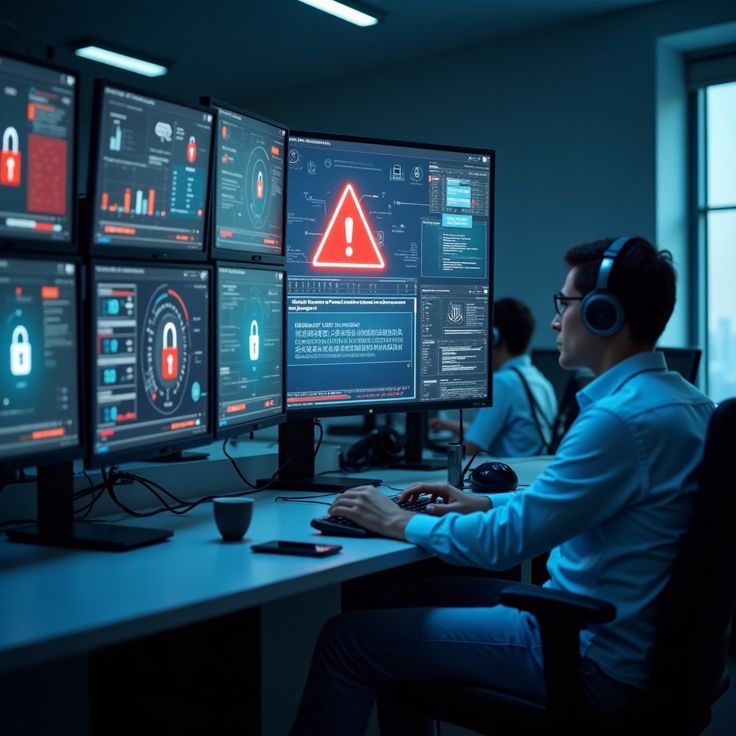
As businesses increasingly rely on digital platforms, the need for robust scam detection mechanisms has become crucial. The debate between Automated Scam Detection AI and traditional methods is at the forefront of this discussion. Which approach is better for safeguarding organizations and ensuring sustainable growth? Let’s dive into the details.
The Rising Threat of Online Fraud
Online fraud has become a pervasive issue, costing businesses billions annually. From phishing attacks to identity theft and financial scams, fraudsters are leveraging advanced techniques to exploit vulnerabilities. Organizations must adopt proactive measures to combat these threats, and the choice between AI-Driven Scam Prevention and traditional methods is critical.
Traditional methods, such as manual monitoring and rule-based systems, have been the go-to solutions for years. However, with the increasing sophistication of scams, these methods are often inadequate. This is where Automated Scam Detection AI steps in, offering a more dynamic and efficient approach to fraud prevention.
What is Automated Scam Detection AI?
Automated Scam Detection AI refers to the use of artificial intelligence and machine learning algorithms to identify and prevent fraudulent activities in real-time. Unlike traditional methods, which rely on predefined rules and human intervention, AI systems can analyze vast amounts of data, detect patterns, and adapt to new threats autonomously.
Key Features of AI-Driven Scam Prevention:
- Real-Time Detection: AI systems can monitor transactions and activities 24/7, flagging suspicious behavior instantly.
- Adaptive Learning: Machine learning algorithms improve over time, becoming more accurate in identifying new types of fraud.
- Scalability: AI can handle large volumes of data, making it ideal for growing organizations.
- Reduced False Positives: By analyzing context and patterns, AI minimizes the chances of flagging legitimate activities as fraudulent.
Traditional Methods: Are They Still Relevant?
Traditional scam detection methods typically involve manual reviews, rule-based systems, and basic analytics. While these methods have been effective in the past, they come with significant limitations:
- Limited Scalability: Manual reviews and rule-based systems struggle to keep up with the volume and complexity of modern online transactions.
- Reactive Approach: Traditional methods often detect fraud after it has occurred, resulting in financial losses.
- High False Positives: Rule-based systems frequently flag legitimate activities, leading to customer dissatisfaction and operational inefficiencies.
- Inability to Adapt: Fraudsters constantly evolve their tactics, rendering static rules ineffective.
While traditional methods may still have a place in certain scenarios, they are increasingly being overshadowed by the capabilities of AI to detect online fraud.
Automated Scam Detection AI vs. Traditional Methods: A Comparative Analysis
1. Accuracy and Efficiency
- AI-Driven Scam Prevention: AI systems excel in accuracy by analyzing complex patterns and behaviors. They can identify subtle anomalies that traditional methods might miss.
- Traditional Methods: These systems rely on predefined rules, which can be too rigid and fail to detect sophisticated scams.
2. Speed of Detection
- Automated Scam Detection AI: Real-time monitoring ensures that threats are identified and mitigated instantly, reducing potential damage.
- Traditional Methods: Manual reviews and rule-based systems often result in delayed detection, allowing fraudsters more time to exploit vulnerabilities.
3. Cost-Effectiveness
- AI to Detect Online Fraud: While the initial investment in AI technology may be high, the long-term savings from reduced fraud losses and operational efficiency make it cost-effective.
- Traditional Methods: The ongoing costs of manual reviews and system updates can add up, especially for larger organizations.
4. Adaptability
- AI-Driven Scam Prevention: Machine learning algorithms continuously learn and adapt to new fraud tactics, ensuring long-term effectiveness.
- Traditional Methods: Static rules require frequent updates and are often outpaced by evolving fraud techniques.
Why Automated Scam Detection AI is Crucial for Organizational Growth
For organizations aiming to grow in a competitive landscape, adopting Automated Scam Detection AI is no longer optional—it’s a necessity. Here’s why:
- Enhanced Security: AI systems provide a robust defense against online fraud, protecting both the organization and its customers.
- Improved Customer Trust: By minimizing fraud and false positives, organizations can build stronger relationships with their customers.
- Operational Efficiency: Automating scam detection frees up resources, allowing teams to focus on strategic initiatives.
- Competitive Advantage: Organizations that leverage AI for fraud prevention are better positioned to innovate and scale.
Real-World Applications of AI-Driven Scam Prevention
Many industries are already reaping the benefits of Automated Scam Detection AI:
- E-commerce: AI systems detect fraudulent transactions, reducing chargebacks and improving customer satisfaction.
- Banking and Finance: AI monitors account activities, flagging suspicious transactions in real-time.
- Healthcare: AI helps prevent insurance fraud by analyzing claims data for irregularities.
- Gaming: AI detects and blocks fraudulent accounts, ensuring a fair gaming environment.
Challenges of Implementing Automated Scam Detection AI
While the benefits are clear, implementing AI to detect online fraud comes with its own set of challenges:
- Initial Investment: The cost of developing or acquiring AI technology can be high.
- Data Privacy Concerns: Organizations must ensure that customer data is handled securely and in compliance with regulations.
- Integration Complexity: Integrating AI systems with existing infrastructure can be technically challenging.
Despite these challenges, the long-term advantages far outweigh the initial hurdles.
The Future of Scam Detection: AI Leads the Way
As fraudsters continue to innovate, organizations must stay one step ahead. Automated Scam Detection AI represents the future of fraud prevention, offering unparalleled accuracy, efficiency, and adaptability. While traditional methods still have their place, they are increasingly being supplemented or replaced by AI-driven solutions.
For organizations committed to growth and security, investing in AI-Driven Scam Prevention is a strategic move. By leveraging the power of AI, businesses can protect their assets, build customer trust, and stay competitive in an increasingly digital world.
Conclusion
The choice between Automated Scam Detection AI and traditional methods is clear. While traditional methods have served organizations well in the past, they are no longer sufficient to combat the sophisticated scams of today. AI-driven solutions offer a more effective, scalable, and future-proof approach to fraud prevention.
For organizations looking to safeguard their growth and reputation, adopting AI to detect online fraud is essential.